Talent is numbers – data science use in sports’ teams
Kamil Skuza
Reading time: 4 min
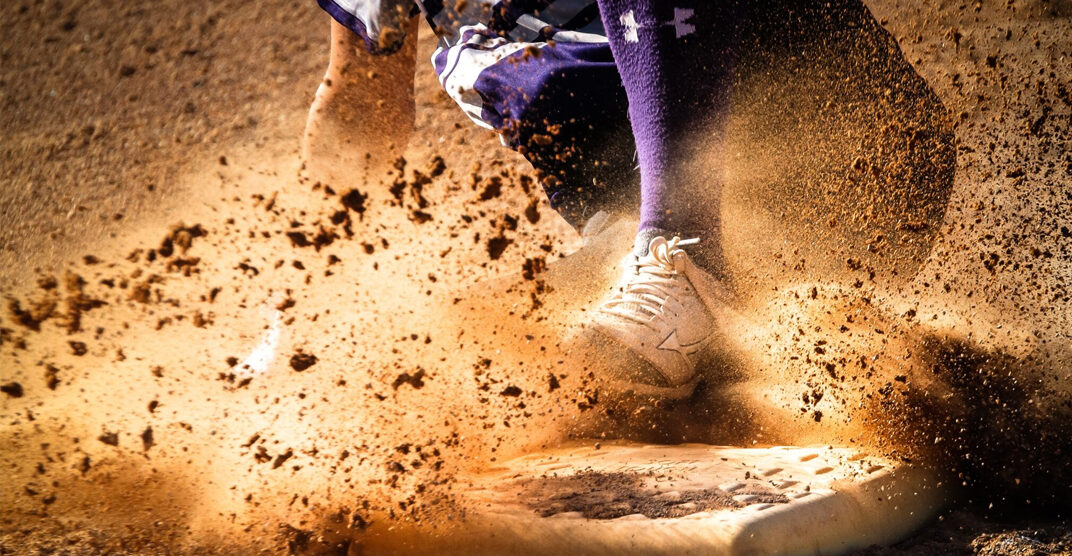
Can you build a perfect baseball, soccer, or basketball team based on statistics? Perfect – no. But winning game after game – yes. How to do it? Not much is needed, just proper implementing, transforming, and visualization of data.
While creating a dream team in a baseball league, talent hunters, scouts, managers, and coaches mainly look for players with high:
- batting average (of hitters)
- the quality of those hits
- number of offensive assists
- stolen bases
Billy Beane, general manager of Oakland’s U.S. Athletics, in 2001, changed the approach to baseball, a sport that had clung to its strategy of forming teams for nearly 150 years.
Billy constructed a new dream team model, focusing on factors such as:
- hits (the number of scored runs divided by the number of possible hits)
- percentage of scored runs (dependent on various variables such as hitting power, defensive mistakes, defender choices, catcher interference)
Where it came from – such a drastic shift in thinking and authoritative approach to the most heavily rooted sport in the U.S.?
First, if you don’t know what’s at stake – it’s money. Oakland’s Athletics team had about 3 times lower the budget of the undefeated New York Yankees ($40 million vs. $120 million), so they couldn’t afford to nonchalantly shop for the most popular players in the league.
Second, during his tours of other teams, in the midst of player transfer negotiations, he met the unassuming Peter Brand, who said behind the scenes that he thought the approach of decision-making managers in baseball had been wrong for decades. Then he uttered the now iconic words: “Your goal shouldn’t be to buy players, your goal should be to buy wins.“
The other day Bill hired Peter, and together they sat down to use Peter’s proprietary software for analyzing massive amounts of data. And, just as importantly, to visualize this data, because without proper processing and preparation it was almost useless.
Imagine 162 games in a season where a total of 30 teams with 25 players clash with each other:
162 x 30 x 25 = 121,500
This gives us a minimum of 121,500 meaningful numbers and statistics (points, bases, fouls, runs, hits, etc.) per season to analyze. Only under the theoretical assumption that each player only makes a throw once, hits the ball once, runs the bases once, etc. So in practice, there is much more data to be extracted from a single season. In addition, Peter also had to take into account at least the last few seasons, since most players were still active in the league.
The statistician had to enter a huge amount of data (millions of rows), extract the most important ones, and, in addition, load and present them in such a graphic way that Billy could understand his reasoning and strategic assumptions for building a new team for the upcoming season.
Peter saw that the entire baseball league was primarily driven by buying players who had high batting averages and high home run rates. His view – rightly, as it turned out – was that all they needed to do was focus on players who had a high rate of getting on base, simply. There were more players like that on the market and they were much cheaper. What’s more, they could still work on their skills by increasing their efficiency in getting on base.
The result? Oakland’s Athletics became the first team in baseball history to win 20 straight games without a single loss. The team also won 4 American League West titles.
What’s more:
- American baseball league player data were publicly available since 1960, but no one was using it properly.
- In India, in cricket, each team has its own analyst for so-called “performance analytics” of players based on 4 major data.
- In NBA, in 2008, 5 teams were using data analytics. As of 2016, every 30th team has a full-time data analyst.
- E-sports has been using data analytics since its beginnings for detailed insights into players’ strongest and weakest points, behaviors, and reaction times in specific situations, locations, and positions where they perform best and worst.
The most important moral, however, is this: Peter had incredible programming, statistical, and highly developed mathematical and logical thinking skills, along with Billy’s business acumen. Today, you don’t need to be the alpha and omega of statistics to make such (or lesser, but still effective and lucrative) change. All you need is the right software and people who are fluent in it. Such as Business Intelligence tools, which are designed to turn business users into “data masters.”
Learn more about data science tools: https://bpxglobal.com/en/solutions/altair/
Source:
- Bibliography:
- Moneyball: The Art of Winning an Unfair Game – Michael Lewis, 2004
- Moneyball – Bennett Miller, 2011
- https://datasciencedegree.wisconsin.edu/blog/moneyball-proves-importance-big-data-big-ideas/
- https://medium.com/@dyutilal/moneyball-the-must-watch-movie-key-learnings-for-every-aspiring-data-analyst-and-data-scienctist-756690e45aa3
- https://capablemachine.com/2020/10/27/moneyball-data-science/
See recent writings
You drive us to strive for excellence in delivered projects and common challenges. Feel invited to read out blog that provides more in-depth knowledge on our implementations and experience. Read articles about digital business transformation, ERP and Business Intelligence systems. Discover interesting practical applications for future technologies.
Contact us!
Let’s talk! Are you interested in our solutions? Our experts are happy to answer all of your questions.